Jagdeesh Prakasam – VIX Decision Trees
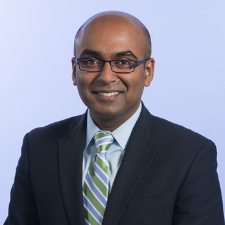
In this episode, we talk with Jag Prakasam, CEO of Rotella Capital Management. Jagdeesh Prakasam oversees all aspects of the firm, including its investment, FinTech solution offerings and operations.
He joined RCM in 2003 as a Researcher primarily focused on supporting the research efforts in portfolio construction, risk management, and overlay strategies for the firm’s core trading strategies. Jagdeesh Prakasam was appointed as the firm’s Chief Executive Officer in 2019. Prior to that he was the Co-Chief Investment Officer alongside Jagdeesh Rotella since 2014. Jagdeesh Prakasam has been managing various portfolios ranging from intraday to intermediate-term holding periods across both futures and equities spaces.
We talk about Jag’s decades of experience in the markets. From doing his thesis in neural networks to being at the vanguard of trend following strategies. We talk about how machine learning has evolved and what are the pros and cons to implementation on vix strategies.
I hope you enjoy Jag’s insights as much as I did…
Â
Listening options:
- Listen on Stitcher
- Listen on iTunes
- Listen on Spotify
- Listen on TuneIn
- Android users can grab our RSS feed here.
Â
Â
Have comments about the show, or ideas for things you’d like Taylor and Jason to discuss in future episodes? We’d love to hear from you at info@mutinyfund.com.
Â
Transcript for Episode 23:
Â
Taylor Pearson:
Hello and welcome. This is the Mutiny investing podcast. This podcast features long-form conversations on topics relating to investing, markets, risk, volatility in complex systems.
Disclaimer:
This podcast is provided for informational purposes only and should not be relied upon as legal, business, investment or tax advice. All opinions expressed by podcast participants are solely their own opinions and do not necessarily reflect the opinions of Mutiny Fund, their affiliates or companies featured. Due to industry regulations, participants of this podcast are instructed to not make specific trade recommendations, nor reference past or potential profits. Listeners are reminded that managed futures, commodity trading, Forex trading, and other alternative investments are complex and carry a risk of substantial losses. As such, they’re not suitable for all investors and you should not rely on any of the information as a substitute for the exercise of your own skill and judgment in making a decision on the appropriateness of such investments. Visit mutinyfund.com/disclaimer, for more information.
Taylor Pearson:
Hello and welcome. This is the Mutiny Investing Podcast. This podcast features long form conversations on topics relating to investing, markets, risk, volatility, and complex systems.
Disclaimer:
This podcast is provided for informational purposes only and should not be relied upon as legal, business, investment or tax advice. All opinions expressed by podcast participants are solely their own opinions and do not necessarily reflect the opinions of Mutiny Fund, the affiliates or companies featured. Due to industry regulations, participants of this podcast are instructed to not make specific trade recommendations, not reference past or potential profits. Listeners are reminded that managed features, commodity trading, Forex trading, and other alternative investments are complex and carry a risk of substantial losses. As such, they’re not suitable for all investors, and you should not rely on any of the information as a substitute for the exercise of your own skill and judgment in making a decision on the appropriateness of such investments. Visit mutinyfund.com/disclaimer for more information.
Jason Buck:
Hi, I’m Jason Buck. I’m the CIO of Mutiny Fund and I’m sitting down today and have the pleasure of talking to Jag Prakasam from Rotella Capital Management, where we’re going to talk about all things, volatility, machine learning, et cetera, how to build actually a very quantitative based research and investing firm. And Jag is coming to us from Seattle today. And how’s Seattle? How’s the weather? How’s life treating in Seattle, Jag?
Jagdeesh Prakasam:
Surprisingly Jason, the weather’s fantastic. And I don’t see a cloud in the sky. This is the best kept secret in Seattle is we don’t want too many people coming. So we keep telling people that it rains all the time. So it’s good.
Jason Buck:
I mean, you spent time in Chicago, but I grew up outside of Kalamazoo, Michigan. And I remember they always had this statistic that we only had like 64 days of sunshine a year. And it was second only to like the Seattle area. So I know exactly what it’s like. So I want to start off basically with your personal history. So during your undergrad, what was your undergrad thesis in?
Jagdeesh Prakasam:
So my undergrad, my background is in Chemical Engineering and my thesis was on the application of artificial neural net on heat exchangers. So I had coded up a C program essentially to apply neural nets to manage heat exchangers. And that was my first introduction, almost an accidental introduction to what is today considered data science. And I was fascinated as soon as I could see that working and the amount of energy cost saving that we could achieve in that. And that got me into thinking, oh, there could be so many different places in the world, so many different verticals that this could be applicable too, this kind of data modeling. And that took me to Chicago to do my master’s in Financial Engineering. And I got into hedge funds and modeling all the time. So that was sort of my beginning into this.
Jason Buck:
I don’t want to date you but how commonplace were neural networks at the time you were working on your undergrad thesis?
Jagdeesh Prakasam:
It was in the late ’90s there and outside of finance I think it was known but it was not as widely used. It was still a theoretical concept but it was still a very slow progression in terms of application. I remember it took us like three years to implement it. I mean, it took me three months to code it up, but it took three years to actually get a sign off on it. So it was a pretty slow process back then unlike finance where you’re eager to sort of apply your algorithms right away and test it across the markets.
Jason Buck:
And probably now you look back and go, man, I was so naive back then and we’ve come such a long way with neural networks.
Jagdeesh Prakasam:
Oh, absolutely. I’m embarrassed. Especially in finance, it’s a different ball game, right? Because due to the nature of the markets and how easily regimes can change and environment can change, the data can change, it’s actually a lot tougher to apply neural nets to finance than it is to conserving energy in a heat exchanger plant. So absolutely. And hats off to all of those who apply neural nets and make money in finance because it’s a very tough problem to solve.
Jason Buck:
Yeah. It’s demonstrates like closed loop and open loop systems and dealing with those. So you alluded to you went to the Illinois Institute of Technology to get your MBA, what was your first job in finance coming out of school?
Jagdeesh Prakasam:
I actually started as an intern at Rotella. This is 20 years ago. And then it was right out of after my master’s, so I started at Rotella and since then I have done multiple roles. I started out as an analyst and went on to be a portfolio manager, managed a whole variety of different strategies in different timeframe, short term, medium term, longterm primarily in futures. I did have exposure to stocks, but primarily in futures. And fast-forward, I become the CIO in 2014. At the end of 2014 and 2018, I took over as the CEO of the firm.
Jason Buck:
Tell me a little bit more about Robert Rotella and the founding of the firm. He has a very storied history and that might give us some insights on how the internal thesis or the thought behind how the firm was originally built.
Jagdeesh Prakasam:
Yeah, absolutely. Either Robert was the original… We think of him as one of the early quant systematic traders. So we have always been a systematic shop. We’ve never been a discretionary CTA that became systematic or anything like that. This was baked into our DNA. We have never made a discretionary trade. We are always following models and algorithms internally no matter what we’ve traded. So he founded, he started out trading. He was seeded by a commodity corp in the early ’90s, 91. And-
Jason Buck:
I really hope one day if I ever have some free time, I want to make a documentary about commodities corporation. Is it one of those-
Jagdeesh Prakasam:
All these traders that came out of it, right? I mean, that’s a huge club.
Jason Buck:
Yeah. A good friend of mine is like a Michelin Star chef and he always talks about the lineage of all the chefs and he tracks them all the way back and they only have like a few branches of that lineage or it’s like I was reading Predator’s Ball again the other day and it’s like, it’s amazing how many of today’s billionaires come out of Milliken’s group back in the day. And so same thing with commodities corporates, some of the legendary traders of ’80s, ’90s, all the way to today, a lot of them came out of commodities corp, and most people don’t know that story at all. And somebody’s got to tell that story because it’s got to be fascinating.
Jagdeesh Prakasam:
Yeah. It is a beautiful breeding that they found the right… Their algorithm of picking the traders and seeding them was I think it’s a success story in itself, how they did it. And Robert came out of it. He was the quant systematic guy. There were a lot of discretionary traders like Bruce Kovner and others, but he was the systematic trader. And as life would have it, he had a phenomenal run from there in the ’90s and early 2000s. And commodity corp was bought over by Goldman Sachs late ’90s and they continued to keep an allocation with Rotella Capital and that’s where the founding of the firm came into being. And so far we operate like… One of the things that Robert’s always sort of insisted that we operate as a research first firm and that’s one of the reasons we have continuously researched in a while, because one of the things was his requirement to us, I remember going back 20 years, was that the day we stop researching or evolving or trying to build a new model, that’s when it’s the beginning of the end.
Jagdeesh Prakasam:
It is one of those things. It doesn’t matter how great a year you had last year or how great a quarter you had this past quarter but you had to continually research and get ahead of where things are. And that’s been a very good sort of thing that continues to remain within the firm across any department you pick, technology or research, they’re continuously evolving. Even today, I can tell you a couple of them are looking at the impact of cryptocurrencies on publicly traded markets. These are all forces in play. And we continuously look at it.
Jason Buck:
And then there was kind of a shift in the firm in 2008 for lack of a better term. Can you sit down and talk about that or the way you guys not changed direction or pivoted, but just started thinking about market slightly differently?
Jagdeesh Prakasam:
Yeah. So see, at our peak AUM, we were at about 1.6 billion at the time. We did very well in 2008. We delivered on the mandate our products were meant to deliver, which was great. But one of the things that we did was we also seeded a bunch of outside traders after 2008 because one of our macro viewpoint at the time was that equities was going to have a bull run. It was going to have a big bull run. We almost called the fact that managed futures was going to have somewhat a sub-par decade. Maybe we didn’t say decade, but the next four or five years because it felt like the way the FED had come in is that it was encouraging people to buy the dip.
Jagdeesh Prakasam:
There was so much impetus to hold the market up and not let it fall down that we felt there was so much liquidity in the market, and it’s all going to go into equities. And remember, luckily we didn’t have a lot of cryptocurrencies then, because otherwise those would have taken off. I mean, one of the most speculative assets would have taken off and that’s what essentially ended up happening. And because of that, we seeded a couple of managers in the single stock space. And we wanted to raise money in that arena. And so what we did is we in some sense… Robert being Robert, given his net worth and the prop capital of the firm, we don’t need money to run the firm. Okay. So because of that reasoning, he actually decided to write a letter saying that he wouldn’t be raising any more outside funds.
Jagdeesh Prakasam:
If you had a reason for being in managed futures and you are an existing an investor, that was fine, but he didn’t want to raise any more money because he felt that there is going to be a sub-par performance and it may not deliver like people want it to deliver. And from a business standpoint, people think of it… It’s a head scratch sometimes, but from his standpoint as an investor himself, as well as a trader, he was being absolutely honest about it, which in my humble opinion, it’s commendable but sure.
Jason Buck:
Well, that answers my question for me, because the first question I had in my mind is like, is you’ve transitioned from being a managed futures firm, right? And then in 2009, you say, actually mentors aren’t going to do well, we think stocks are going to do well. Usually investors are like, I don’t want to hear any of that. Just stick to your knitting. This is what I hired you for. Just do that. And so the intellectual integrity for Roberts will do that, but because he’s sitting on the base of a prop firm capital that’s what allows you to make that transition to go with your gut on that, otherwise, if it’s just totally outside investors, you’re likely to lose pretty much all of your AUM in a scenario like that.
Jagdeesh Prakasam:
Absolutely. You’d shut off. See, one of the things about putting your family name on the firm is that you don’t have the luxury of just shutting shop and starting a new fund. That is not what we do. So we are very careful also internally when we manage money, we always seed our strategies with our own prop capital first to see how it works for a couple of years before we go out and start raising money for it. So that’s something we do. And you’re right, the luxury is because of having that prop capital, we are able to do it. But that sense of honesty and integrity has always been the case. And also it’s driven from the fact that Robert has his family name on it which is important.
Jason Buck:
So you guys started seeding equity investors in-house in 2008, how has that evolved in the last 13 years? Did you start transitioning back out of equities, more into managed futures or other strategies or how did the firm evolve since then?
Jagdeesh Prakasam:
So after that what we did is we had a market neutral strategy that we spun out from Rotella. And I think they did well. They were about three, 400 million, I think when they were spun out. And since then actually to be honest, we have kind of come back to manage futures. So a lot of our strategies, we actually are very bullish on managed futures right now. And we’ve actually put all of our risks capital on managed futures, and this has been a transition for the past couple of years. We’ve been doing this for like three years. And also the quality of research I think we feel extremely confident of the quality of research that we have internally because some of the models and the model basket that we’ve used is way different than what it used to be.
Jagdeesh Prakasam:
So, for example, in the past three years, 70% of our risk is in new research. It’s not from legacy research, which gives us a lot of confidence. We see why it’s outperforming, why we can actually extract alpha in the space. And we’re outperforming the benchmarks substantially. Late last year we outperformed the CTA index by 20 plus percent. So, I mean, there is a reason for it. And I think we are able to stay ahead of the curve. I think people are going to catch up and that’s perfectly fine, but I think we are ahead of the curve is my feeling on that.
Jason Buck:
And then you guys have a panoply of strategies now, but part of that evolution was, did you stop seeding outside investors and now you seeded all internally so basically you’re creating your own commodities corp now, or how does that work?
Jagdeesh Prakasam:
You could think of it like that, but it’s a very collaborative approach. So it’s not… You see, commodity corp had these sort of individual traders and bubble. So Robert knew what Robert was doing, and Bruce Kovner knew what he was doing, and I don’t think they exchanged notes, but the thing is here, it’s way more collaborative approach because one of the risk factors of for an investor coming in now that we’re back out trying to raise money for these strategies, we want to make sure that it’s a stable investment team, because otherwise, if it’s just pegged only to an individual PM without a support structure, the risk is too much on that individual PM.
Jagdeesh Prakasam:
So from both standpoint, from an innovation standpoint, it’s great for us from an investor standpoint, it’s great for you because you have a stable backup team that you know that there is an investment committee discussion every day from 3:00 to 5:00 in the firm. And I can tell you that it happens every day. But so everybody discusses their issues, their problems, what they’re seeing in the market. And roughly everyone has an understanding of what the other program is. And at least two people have an understanding of exactly what the code is in place. So something like that. So there is a redundancy as well built into that structure.
Jason Buck:
So I’ve been on one of those investment committee calls with you, and there is a lot of people on those. And so part of that is you have experts and researchers all over the world. And so are you going out and looking for them, or are they coming to you with strategies? Or how do you guys kind of put that to you?
Jagdeesh Prakasam:
So typically what we do is I’ll tell you a recent example. We wanted to build our own natural language processing engine. So what we did is we were trading sentiment signals for a couple of years, but what we found is all these art data providers that we were looking at were bringing in their own sort of natural language processor and coming up with a sentiment signal, whether they’re bullish on the S&P or bearish or bullish on any individual stock or bearish. So what we were doing is we would aggregate across all these stocks and come up with one signal for S&P futures or NASDAQ futures, or what have you. And futures being a 24 hour market, we were capitalizing on the fact that we could make a trade before the cash markets opened. Okay. So that was where our play was.
Jagdeesh Prakasam:
But what we found is there was too much risks with sort of outsourcing your natural language processor in that sense, because the mandate for the provider is very different than ours. They don’t know what we’re using it for, and they could potentially change it at what rate and we had to write in things like we wanted a fork and we wanted to maintain it, still it’s not the same. So what we did is we built our own natural language processors. So when we went down that path, so we had an objective and then we hired around it. So we looked for researchers around it. We looked for people who are involved in it and that’s how we sourced candidates.
Jagdeesh Prakasam:
And it didn’t matter where they were. Like you mentioned, we have a researcher in Scotland. We have a researcher in the UK. We have research in India, we have a researcher in Italy. We have people in Seattle, the majority of them sit in Seattle, of course. And with the pandemic, of course, I guess it doesn’t matter where you sit, but what we have done is we have looked for talent to come up with the best idea possible who can achieve it, and they necessarily don’t have to be in finance. It’s fine. As long as they had an expert in that area, that’s good enough for us.
Jason Buck:
That begs an interesting question. One of my favorite business books is called Loonshots. And it’s about the combinations of different kinds of cognitive diversity that you need in a company, but also the tension between those. He calls them like artists and soldiers and how they don’t think alike, but you need them to work together to solve problems. So it’s like if you’re searching for somebody that has a deep expertise in machine learning, how do you combine them with somebody like you who has a deep expertise in financial markets to make sure that it actually can work or overlay with the actual financial environment and how do you put those together because it’s a really delicate balance.
Jagdeesh Prakasam:
Yeah. That is where the art part of the business comes into play. You’re right because you have incredibly smart people who know the theory behind it but haven’t applied it to finance. And they tend to look for black and white. I’ll give you a good example is everybody talks about machine learning models, but when you talk about machine learning models outside of finance, they’re looking at use cases where the signal to noise ratio is really high. The potential margin of error is low, the possibilities are low, and they’re applying it to those use cases. And everybody’s fascinated looking at it. But when applied to finance, it just blows apart the number of scenarios that this particular algorithm should be prepared for.
Jagdeesh Prakasam:
And that’s something I can tell you people who come from outside finance just don’t like it. They look at it and say, “Ah, you’re asking me to make a black and white decision in a grey market.” And I’m like, “Absolutely, this is exactly the game. We just need a slight edge and we are going to have losing trades.” And the idea of losing trade scares them. But I said, “Yes, we are absolutely prepared for this.” We know that ahead of time this is going to happen, but we are looking for an edge where we are trying to increase the odds. We are never going to look at a trade and say, this is a sure shot. It’s not like a pure arbitrage trade here that we know ahead of time that this is going to be a profitable trade.
Jagdeesh Prakasam:
So that’s always a challenge. So what we do is we tend to pair them up with people who have been in finance within our firm for at least a year or two before they start building models on their own. So they get to kind of watch alongside… They have a… One of the first things we do is we put an intraday P&L dashboard on their desktop and say, “Just watch the markets.” Here are the trades we made today, or here are the trades all these different strategies are doing and watch in real time. I remember one of my favorite hires earlier and was camping and I think you’ve met him. And he came in and we watched the May 6th, 2010 meltdown live.
Jagdeesh Prakasam:
We were watching the screen as it was happening. I think it was just before around 1230 PST, and we were watching this happen and we were trying to see, oh, how would our models do? I mean, it recovered quite a bit that day, but I remember that it suddenly got them hooked on to watching the market. So there could be so much action. If you remember, 2009 was God boring. After March of 2009, it got boring. It was kind of this low volatility upward grind kind of thing.
Jagdeesh Prakasam:
But once that happened, it got the pulse going and saying, “Okay, wait. So these are all scenarios that can happen even intraday.” And actually we landed up building a whole variety set of intraday models. And we always kept that as one of the scenarios that could happen. And we would throw that in at different points to see would the model select. We would sort of make fake data by trying to throw that event in and see how would the model react. So that’s always an education process, but that’s how we’ve kind of tried to get it in, but it’s definitely an art. There’s no formulaic approach to this.
Jason Buck:
I’m sure the first thing you have to teach them is about trading costs, right? That the best algorithm in the world usually gets destroyed by trading cost. And that’s mind blowing alone is like slavery in Egypt, bid-ask spread and commissions.
Jagdeesh Prakasam:
I remember even myself this is going back to ideas and I’m going to embarrass myself, I made my first trading model and it was like typically I was doing absolutely research for the most part for trading models. And finally, I got down to the point where I was building my own trading models and one of my first models in the first week this happened, when I got at the time like two sharp or something and I was so kicked. I had to take a walk.
Jagdeesh Prakasam:
I remember I was here in Washington on Katelyn Point, and I kind of took a walk out because I couldn’t believe it that I would have an idea two sharp system. And I am terribly embarrassed of course. I walked, I got myself a Starbucks. I came back, I had to think about it. And then I said, wait, I don’t think I have added slippers and transaction costs into this thick. And once I added it, it dropped so badly. It was like, man, I couldn’t… And it was a short term system as you can imagine with short term systems more so than longterm systems, it can kill you.
Jason Buck:
Yeah. I have very embarrassing stories. And we’re going to talk about VIX today. So I’ll talk about that. It was like almost a decade ago, I was developing all these intraday VIX arbitrage models and everything, and they were so beautiful, but then they couldn’t hold up to the transaction costs, especially that $50 bid-ask spread that you-
Jagdeesh Prakasam:
Oh, the list is huge.
Jason Buck:
So that is embarrassing as well. It does make me question like Renntech, have you ever had to use a human override and pull the plug where you felt like, oh, there’s just something outside of our algorithms here. Let’s just take risks down dramatically and then basically shut down the algos for a day or two or a week, or has that ever happened to you guys?
Jagdeesh Prakasam:
No, we have never shut down an algo like that. What we have done though is turned down leverage and it has happened very infrequently. Especially I would say when even in 2008, if the uncertainty level really went outside the norm of what we had tested these models for, we would turn down the overall leverage a little bit, but we have not shut down the model or not traded for a day or anything. That’s the beauty of it. Funnily enough, we have never stopped trading. We have always had a trade in the market for 30 years now. So that we have not done, but we have turned down the average, but we try as much as possible not to interfere in the model because we believe that our techniques have tested them, but unless it got really uncertain like when it becomes the odds become a coin toss between someone doing something in Washington to save the day kind of thing, then you kind of think about it.
Jagdeesh Prakasam:
So we might turn down the wall a little bit, but for the most part, we haven’t interfered with the models in the past. I mean, in the past decade, very rarely, if that, because the reason is we’ve actually figured out better ways to hedge the portfolio. Ideally, it’s counter-intuitive. Ideally what everyone wants is to have passive products that’ll hedge you, but these passive products themselves are dynamic in nature. They are really actively traded products that they just want to hold on to it and not worry about the exposure because no one likes market timing and no one likes prediction, and they haven’t really succeeded there. Very few people have succeeded and there almost no one was succeeded for a long time.
Jagdeesh Prakasam:
So that’s always been the case. So at the heart of it, we have found better ways to come up with products that will hedge actively traded products that will hedge our exposure. So we don’t have to make these sort of decisions and allow the strategy to kind of do its thing. And it’s actually really informative. Even if it had a drawdown, but it was hedged, that’s actually a very educational thing from our standpoint. So we have stretched the limits. So you as an investor could know, okay, this is a case that’s gone and come back, it’s recovered. It didn’t kind of collapse and never come back. That’s the risk you have.
Jason Buck:
Exactly. So a primary hedge is like VIX products or long VIX products and we’ll talk about that. And then how what’s interesting about those hedges is you may go years without getting a sample size to even look back and go, “Oh, I have it here,” which is tough. But just as a quick primer typically the VIX and the S&P are inversely correlated over time and due to variance. But most of the time the VIX term structure is in what we call contango where the future months are subsequently higher than the current front month. But then when you have a spike or a sell-off in the S&P, the VIX is going to spike through the roof, like in March, 2020, and then the curve goes into backwardation where maybe the front month or second month are higher than the few future months out in the curve.
Jason Buck:
So we have these contango and backwardation environments, but then from like July, 2020 to now, we had these medium vol environments where like the vols surface is kind of flat in the VIX curve, or you have a kink in the curve due to the election cycle. What do you guys think about that new normal? How do you guys assess that? And whether it’s different from a contango or backwardation, it’s kind of a different environment.
Jagdeesh Prakasam:
Yes. So that is one of the… So I’m going to pull back a little bit. I’m going to wind back the clock in terms of how we arrived at this model a little bit too, because we have always been in the space of alternative investments even with our managed futures products, the classic trend-following product, what have you, it’s meant to provide crisis alpha. Ultimately, what are we trying to do is when the equity market drops, when the stock market drops, can we provide a hedge? Can we be positive? Can we take some risk off of the books? But while the market isn’t a bull market, we want to spend the least amount on insurance. So that way, the buyer or the investor feels like they’re getting the upside and they can sleep at night knowing that if tomorrow the market crashed, they were hedged.
Jagdeesh Prakasam:
So that’s the utopian sort of framework in which all sort of alternative investment managers work in. They’d love to sell the pitch that is almost 99% of the pitches that you will see, which is always benchmarked to the stock market. But what we found earlier on with our trend following, which did phenomenally well in market crashes was that we realized that even though people like to sell it as a crisis alpha strategy, it’s not targeted to be a crisis alpha strategy. It is targeted to be an absolute return strategy, which happens to diversify you on the tails, but it is not a given. By simply having it, you cannot assume that it is going to diversify you every time the S&P crashes. The classic example is right now, when the bond deals are going up, it is going to be interesting.
Jagdeesh Prakasam:
If you had a crash, how would the CTAs do? Okay. Last March, the trend followers to find our product did really well. But again, because the bonds went up, the yields dropped, that’s fine. We were able to provide the hedge, but again, going into this environment where we see the rates going up and the use going up, it’s going to be a difficult one to pull off because we don’t see much sort of movement in the bonds in terms of how it can do us fine, right? So this is something we realized several years ago, where we were thinking about this exact situation, where we were saying, what is a direct way to provide that hedge for the S&P? Ultimately if you have anything over 0.7 or 0.8 beta to the stock market in your portfolio, you want to hedge and you want to bring it down a little bit.
Jagdeesh Prakasam:
And how do we give you that hedge? So what we decided was to do a long week strategy, but I’ve heard some of your podcasts and your whole platform is based on this. So in some way, I applaud you for building something so focused that it actually captures something that I haven’t seen with a lot of allocators that I’ve spoken to. I mean, the way you put together the platform with different managers in them, it’s actually a phenomenal combination and I can see value in doing it the way you have done it. And so the idea in which we went about this is we said, okay, we wanted to have a long VIX strategy. So we never wanted to get caught being shot wall in a market crash.
Jagdeesh Prakasam:
And because that is a temptation. When the S&P’s sharp is over one from 2009 to 2019, March or Jan end this is what happens is that the longer you go without an earthquake, the less people care about having an insurance policy for an earthquake. And eventually all these people who provide returns… And this has happened with the other CTA managers as well, because they have an absolute return mandate. Their objective is to maximize revenue and fees. So at some point, their strategy starts going away from the stated mandate. And I don’t blame them. I mean, it is what it is. But in this particular situation, a lot of the world managers started putting on new models that were going sharp wall because they wanted to capture the beta to the stock market.
Jagdeesh Prakasam:
They wanted that upside returns in the stock market. They wanted to put it in, but we always felt uncomfortable about it because fundamentally when someone invests in a wall product, what are you thinking of? It’s going to diversify me when the stock market crashes. That’s the fundamental requirement. Everything else is better for all in my book anyhow. And the other part is I shouldn’t bleed too much when the markets are up in a tier. So that’s the second most important thing as well. So what we said is let’s remain long wall all the time, never sharp, but then come up with a hedge with a long equity hedge. So that’s where we said we’ll go long S&P futures and long VIX futures. And in a situation like due to the asymmetric relationship between the two, anytime the stock market crashes, the wall appreciates a lot higher.
Jagdeesh Prakasam:
So we would lose a little bit of money on the S&P leg, but we are going to make a lot more money on the VIX’s leg, therefore, net and net will provide positive returns. But on the flip side, if the S&P is up on a tier and we have a strong contango as I call it. So the curve is a little more steeper, then actually the beauty of that environment is this that since we had the long SQ position, we make money on the equity leg, but we can hedge it with a very small quantity of long VIX, which is good, because those are the environments where if there is a crash, there is an outsize moment in VIX. So it doesn’t matter how small the position it will cover for that leg.
Jagdeesh Prakasam:
So that is how it was structured. But here is an environment you will walk into. There are risks in the strategy like with any other strategy is that if you get into sort of a flat wall phase or a medium to high wall, and it continues to remain there, and then you have a market crash, VIX is not going to do a whole lot. Okay. Unless this is a huge market. It has to be a huge market crash for VIX to do something. If it is a mini crash like a 2% down day in the market, it’s debatable whether VIX will do anything. And those are the environments where it’s going to be a little challenging in strategies who are set up like this. However, thankfully these phases don’t last very long. So that has been proven time and again. So it remains to be seen, but as also that that’s the… It remains to be seen, let’s see how this kind of goes.
Jason Buck:
Yeah. So there’s a couple of things I want to unpack that you said in there. One is, if you think about managed futures historically, managed futures also being commodity trend-following, so at one point it was marketed as crisis alpha, even though maybe a lot of managers didn’t want to market it that way, but they were happy to do that after 2008, because 2008 was more of a slow long drawn out recession and mandate futures trading incredibly well in that environment. And so that’s where they got that crisis health for the moniker. But what they all know all too well is that managed futures or CTA trend is actually uncorrelated. And so a lot of people miss the meanings between correlated, uncorrelated and negatively correlated, and that’s what you’re hinting at is with VIX or put options, you want to be negatively correlated to the S&P.
Jason Buck:
When you’re uncorrelated, that means you can move in tandem with the S&P, it’s more of a random noise or process. So one thing I want to pack about this is why you’re looking at VIX systems, because they have that negative correlation. The other piece to it and thinking about it is as you alluded to, I just want to highlight it is that when you’re in a contango process and your long future fixed contracts that roll down is killing it, that’s bleeding you out, and that they can bleed to death, right? So this is why you want to hedge with the long S&P or the ES futures is to help cover some of that bleed. But as you alluded to, when there’s a sell-off, VIX will spike, and it has much more convexity than the S&P does.
Jason Buck:
So therefore, it’s all about your hedge ratio. So with your guys’ machine learning long only volatility program, it’s all about the hedge ratio at the end of the day. And I think what’s also interesting is not only are you guys using the VIX and S&P, you’re using the VIX stocks and the Euro stocks, so you also are trading the European stock market. And then there are subsequent VIX product as well. But at the end of the day, it all boils down to the ratio, right? Is how do you get the ratio right between how many VIX contracts you trade and S&P contracts and then combine that with the steepness of the contango in the market or the backwardation or this medium environment we’re in where you’re just getting kind of turned away for a little while.
Jagdeesh Prakasam:
Right. So internally the former ratio… I’m going to talk to around frameworks, because this is something that we use in the trend following portfolio. We do this with a mixed portfolio as well. So we have two different… We have a neural network framework, and then we have a random forest framework. Okay. So both of them are supervised learning systems. What I mean here is essentially we have an objective that we want to achieve, and we have a multitude of inputs here. And during the training phase, we figure out are these… We optimize these models to make sure that it’s meeting the objective, and we continually train these models. And with respect to the wall strategy that we are discussing with VIX and S&P, it has a neural network framework in it, which essentially alters the hedge ratio.
Jagdeesh Prakasam:
It’s almost like a position sizer. So in this strategy, we are not trying to predict when is the next crash? That is not the objective. The objective is all we’re trying to do is we want to pay the least amount of insurance to protect the long SQ position. But when the crash does happen, we want to hold onto the gains because a lot of strategies might be right. This is one of the things that happens with the long VIX strategy, which is not hedged right, or when the hedge ratio is off is they give back all the returns. Typically, if there is a steep crash, which recovers even midway, halfway through the next day, you will give back a lot of the returns, right? And so our goal is not only to pay the least amount of insurance, but to also hold on to that payoff whenever it happens.
Jagdeesh Prakasam:
So those are the two sort of objectives we have that we are optimizing our models for. So you can use a multitude of different models. If you’re building a model today, take your pick. You can use any algorithm, but what’s important is the objective function that you’re trying to achieve and to see on a continual basis, are you achieving it because that was your objective. So that’s the beauty of the machine learning framework that we use is you already know ahead of time, it’s easy as an investor to know, okay, what is it that they’re trying to achieve and has it achieved that?
Jagdeesh Prakasam:
It’s a good way for you to evaluate clearly rather than an unsupervised learning system where they don’t have an objective, but they will say, let me find patterns in the data. And it’s an evolving sort of hypothesis. And that’s a tough one, because then as an investor, you don’t know what… I’m not sure if the builder themselves know as to why something didn’t do well or something did well, but granted, they’re trying to look for new patterns as they want. But this is a more controlled environment from that standpoint.
Jason Buck:
It’s such a subtle thing that you’re saying that I want to really highlight it is that like, when I had a lot of people outside our industry think of machine learning, they think it’s just unstructured. It teaches how to trade. It tells you when to go long or short. But that’s not what machine learning is good for. It’s good for targeting into a very niche concept. Like I think about Dan Rasmussen in Verdad, when they’re doing like their equity investments in small caps, they train their machine learning on which companies are likely to pay down their debt service, not what are the best companies to trade, right?
Jason Buck:
And so you need a very niche qualifier to think about the trade structure, not unstructured of how do I go long and short. So you’re saying you’re using this neural network to think about the hedging ratio solely between VIX and S&P. Did I put words in your mouth?
Jagdeesh Prakasam:
Exactly.
Jason Buck:
Okay, great. So talk to us a little bit about that in this program like you said using neural network and another program using random forest. So kind of talk to us about how the neural network works to kind of move things down the funnel to eventually get to a trading decision on that hedge ratio.
Jagdeesh Prakasam:
So what it does is it uses a multitude of different inputs. The team that built it would probably be more qualified to talk about exactly what their inputs were. I conceptually understand what is going on in that. So I do my best to give you seashells.
Jason Buck:
There’s like 2000 inputs to it.
Jagdeesh Prakasam:
Yes. There’s a 2000 input. One of the challenges is most people think… I know that when people think there are 2000 inputs, is this going to be a rough… Is this a co-fitting exercise? This is the overfitting exercise. That is the first sort of a feeling people have around it, but that’s really not the case. If anything, we are actually reducing the co-fitting aspect of it because what we are doing is we are essentially creating weak learners and ensembling different inputs, so that we are not just relying on one that is ultra fitted to a certain data path or the price path, or what have you. We are actually looking at a multitude of inputs, multitude of dimensions across different datasets, which come from the same distribution. So the distribution is the same, but we are looking at multiple price paths within that on which these models are being fit so that there is considerable level of wiggle room in terms of what sort of environments it can see.
Jagdeesh Prakasam:
So that in itself what it does is it arrives at, okay, so this should be… Ultimately it boils down to this is the X number of contracts we should be in S&P and X number of contracts in VIX based on the profile of the term structure of the VIX. If it’s a steep contango, great, we will hold less number of VIX contracts, a lot more S&P contracts, but yet we are hedged. We also pick up on the upside of the S&P and if there is a crash, that’s perfect. It plays out like we want it to play out. So that’s really the decision buying where it boils down to is that how do you come to that point, where what is the hedge ratio? What’s the exact number of contracts do you want to trade for S&P? What’s the exact number of contracts you want to trade for VIX?
Jason Buck:
So as the system is reducing that dimensionality and you’re getting down to the actual hedge ratio, how often is that getting updated?
Jagdeesh Prakasam:
So this updation happens, I want to say about… It happens in real time in the sense that every new data point that comes in, it happens in New York time. But one data point, one day of data is not going to dramatically change the weightings or the weightings of the neural net itself. It needs considerable amount of data, but it’s taken into account. So you will see that getting optimized over a period of time. But in terms of the scope of the playground that it can play in is considerable unless there is something completely new concept, a completely new concept there and that causes a change in the fitting of the neural net itself. But the neural net itself changes very slowly. I mean, the optimized system itself probably changes once and I don’t know, I want to say once in six months is how frequently it happens.
Jason Buck:
But how often or how frequently are you updating the trading positions or the levels of the ratios?
Jagdeesh Prakasam:
Oh, that happens on a daily basis. So sort of the position that change happens on a daily basis. So we continuously look at the markets and adjust given where it is today, where the S&P is, where the VIX is, we do adjust it every day. So everyday you will see a small amount of physicians either go up or down, but always long. You’re always going to see SQ and VIXs long but it continuously adjust to that market. But when I say in terms of the neural net itself being re-optimized, what I mean is if there’s a dramatic market regime change, this has the ability to kind of adapt but that has to be a substantial market change. But it pull it up. It’s not that it’s not good.
Jason Buck:
But that begs an obvious question. And because you’ve been in this game a long time you know that the neural network is only as good as the data that it’s running on. So it’s like we could have VIX run to 200 next time and we haven’t seen that in the data before. So how do you think about hedging out that risk of it? It’s not in the prior data, but the future real life event could be much greater than we’ve ever seen in the sample size that the neural network is using.
Jagdeesh Prakasam:
Yeah, sure. One of the things that we think of it in case of this particular strategy, that is an issue in some of the other types of frameworks that we’re working on, but the issue, here we are not as worried about it, because it’s the relative relationship between VIX and SQ. If VIX goes to a 200… Or let me put it this way, if VIX drops dramatically and the S&P crashes by 10%, now, that’s an environment we haven’t seen. And sure, we are going to be in a little bit of… We haven’t seen that the model may not handle that well I can see that. The probability of that happening is extremely low but that’s the type of risk I’m talking about. As long as the relative relationship still remains intact, it’s okay. It actually doesn’t matter VIX goes to a 100.
Jason Buck:
Yeah. Well, that’s the best part is people don’t think about that you have an inverse is like when you’re long volatility, those outlier events are only beneficial. Like if VIX it’s 200, year long VIX, so it’s fantastic. But it begs a reverse question is that when we saw March, 2020 with VIX in the ’80s, or even if we saw VIX in the 100s, now you’re developing air pockets underneath a long VIX position. I mean, you’re trying to get your head ratio right but how do you guys think about that like in these kind of 80 VIX environments, it’s kind of a new regime and are you lightening up on position sizing in those scenarios or how do you guys think about affecting the position?
Jagdeesh Prakasam:
Right. So we will lighten up on those environments. But the catch here is this, if there is a drop, it has to be a big drop because in SQ there is going to be a flat faced kind of thing. It may not make much money. It won’t lose much money, but it’s going to be sort of flat if VIX is really high and the markets are also high because the problem is the many crashes, it’s going to be sort of flat. So that’s what you’re going to be exposed to. But definitely if the volatility is high, we are lightening up on our positions because there’s enough wall to hedge. If it has to be a big enough move for it to kind of hedge you on the VIX position.
Jason Buck:
Because did you say-
Jagdeesh Prakasam:
I mean or the SQ position.
Jason Buck:
Yeah. Well, it’s even harder to say if we move from a VIX environment of 10 to 80, like when we’re always in like you’re saying those low vol environments always precede the high vol environment when everybody gets rid of their long volatility positionings, right? So you can make an unbelievable convexity. But then the problem is like when we’re in these mid vol environments and it’s kind of the vols picked up and you’re maybe in the 25, you don’t have as much complexity if it goes from 25 to 40.
Jagdeesh Prakasam:
Right. Exactly. So in an environment like that, we would load up a little more on the VIX positioning because you would need more because the convexity drops, right? So we would need a little more to make up for it. But that’s where the balance is because we have to be careful that we are not overloaded because the problem is if you had these many drops of like 1% days or something like that, the weeks is not going to move as much. And truth be told, if there’s an investor who is trying to hedge their fine date beta to the stock market, I think a 1% drop is okay. It’s not an event that needs to be hedged in my opinion. But if there is a 5% market crash or tomorrow something happens, that’s big. You want that…
Jagdeesh Prakasam:
These strategies are great to protect the destruction of your compounding equation. It’s great because those other big crashes are the ones that will destroy your compounded returns. Also because of the behavior of investors, because they will redeem. About half of them might redeem from their positions when there’s a 10%, an 8% drop in the markets, they’re going to redeem at the worst possible time. And no matter how that curve looks and the recovery looks, they never got anything of that recovery. And then by the time they get back into the market, again, they’re late to the game.
Jagdeesh Prakasam:
So their compounded return is so bad. So instead if they had this insurance product in place, they don’t have to do much. They can hold onto these scenarios where there’s a drop of eight to 10% because they are hedged. So that’s the beauty of these products and all of the products, I think, which are part of the Mutiny umbrella, I’ve had a chance to look at some of them. It’s great. I mean, I see the profile. Kind of without talking to you I can see why you were attracted to that or you wanted it in your stable, right? I mean, I get it.
Jason Buck:
Yeah. We’re always looking for different wrinkles and different strategies and trying to include that in our stable. But what you were alluding to there and you actually said before, and I should have pointed it out is like, it’s interesting. It’s like we’re looking for what these long volatility insurance like strategies, that negative correlation to S&P. But if you ask another question, we have to dig a little deeper, that’s not quite accurate, right? What we’re actually looking for is in a trending up S&P environment, we actually want to be uncorrelated or slightly correlated to S&P and then the sell-off, we want to be negatively correlated, because if I look at the scatterplot of like your machine learning one only, that’s exactly what you’re trying to do, because as you said, you’re trying to maintain a flat or slightly positive carry during a risk on cycle, but then switch, be that negative carry convexity that’s negatively correlated to the S&P in the sell-off, is that a fair way…
Jagdeesh Prakasam:
Yeah.
Jason Buck:
Because you can’t say just negatively correlated because you needed to carry well as well, right?
Jagdeesh Prakasam:
Hey, I love the upside correlation. I think everybody loves the upside correlation. It’s the downside correlation that we’re all worried about it. And most people don’t think of it in those terms. When we talk about correlation, it’s an overall terminology, but in some sense, you’re right. That’s exactly how I would kind of-
Jason Buck:
Yeah. And it’s always that people always bring up correlation, I’m like on what day, right? Correlations is a static picture and it’s not telling you a full story. And so that’s what you have to think about is like, I have a product that has a structurally negative convexity and asymmetry to it. But if it’s also during a risk on cycle, if it has a positive correlation, I’m fine with that because I’m sitting on all this convexity for a sell-off, right? And then you hinted the random forest model. So you have your Rotella tactical long bias volatility program that’s your random forest model, but it’s a rule-based tactical approach. And so what do you guys kind of mean by that?
Jagdeesh Prakasam:
Yeah. So that’s a tactical approach and actually that one doesn’t have the random forest models. The trend strategies have the random future models.
Jason Buck:
Okay. I get it. We’ll get there.
Jagdeesh Prakasam:
But so in that, the VIX curve is played a little differently but there it’s a combination. So it has components of the profile. So that is a classic example of it doesn’t matter what algorithm you’re using, a neural net or machine learning or a decision tree model but what is the objective you’re trying to extract. And that also tries to play off of exactly the same thing. It’s approaching it differently, but when you see the underlying objective, it’s trying to essentially say, I want to reduce the insurance premium. I want to make money on the… I want to be correlated on the upside. I don’t want to be correlated… I want to be negatively correlated on the downside. And that’s really what the objective there is explicitly.
Jagdeesh Prakasam:
And what it does is it reduces risk. So even there, it plays off of this the differential between what the decision tree model does there, and the neural network model does here is they approach the hedge ratio in a different way. But again there again, they are trying to control the wall positioning and the S&P positioning in a way that there are certain times when that one can go totally zero. Like it may not have that hedge so that it feels there is no value. And so in an environment that we have seen in a flattish when the VIX is meant to flat what that will tend to do is try to lean into the S&P position a lot more and keep a really low exposure to VIX in that sense. Because it feels like there has to be a big enough crash for it to make an impact.
Jagdeesh Prakasam:
So that’s the odds. So there is a… Funnily enough if you see the correlation between the two, they are actually uncorrelated. But the objective that they’re trying to achieve is the same. So there is a phase there and I’m trying to think it was in I think Q4 of last year, October or November, where we were in a similar situation where I kept looking at that the tactical program was making money while the machine learning long wall program was not. And I said let’s take a look, dig a little deeper into that. And it was very evident then that what we found this environment, where we can actually lean more so the tactical program was taking a lot less risk on the VIX and taking a lot more risk on the S&P. But again, it was not trying to be cut short VIX, but it still wants to have the same objective.
Jason Buck:
So thinking about that tactical approach, it’s using relative strength or signal to noise ratio to enter those positions, but also it’s going… Like you said, sometimes it can be flat or it’s going long, short based on that term structure. So especially if the term structure is flat, that’s hard to do. So how does it make those kinds of decisions? What does that mean for relative strength or signal to noise ratio to enter the trade? What does that kind of mean and the way you guys think about it?
Jagdeesh Prakasam:
Yeah. So if the signal to noise ratio is really high in the equity markets, it feels a lot more bullish about the equity markets. I see at the heart of it what we are trying to do is if we think the odds of persistence and momentum in the equity markets is high, then we don’t want to be over hedged. So it’s trying to reduce that component. But if the… And as you know, when you’re in a long VIX position in a contango, you have this headwind from the roreals. So how do you make up for it? At that point, you go the long shot track on that to extract that characteristic. But once it’s backwardated, you don’t need to have feature enabled.
Jagdeesh Prakasam:
So it tries to capture some of the payoff, some of the insurance premium that you pay for holding long this hedge in that way. It kind of makes up from a different direction. The ML long only world never does that, but this particular one does it because it tries to pay off that cost that you pay with that feature when you have this headwind. So it kind of capitalizes on that.
Jason Buck:
And then as you alluded to it’s these two strategies are uncorrelated, but in a sell-off, you want those correlations between those strategies just to go to one or negative one to the S&P. And typically that’s exactly what we look like across our whole platform. We’re trying to find managers that are fairly uncorrelated, but then their correlations go to one, which is negative one to the S&P and the sell-off. So it’s very similar concepts is that’s exactly what you’re kind of looking for because then you can kind of hedge some of the volatility between the strategies if they’re uncorrelated during a risk on cycle or other parts of the cycle. Is that the way you kind of look at having two versions of attacking it?
Jagdeesh Prakasam:
Yeah, absolutely. Because especially on the downside, because this is a hedge, we want it to have a correlation of one between them, right? And a negative correlation to the S&P because one of the things as we know like market neutral strategies, for example, a majority of them, there are some that do incredibly well, but majority of them what had happened is they always talk about being uncorrelated to the stock market. And it’s great when the market is up and what happens is when the market falls, what we have found is since majority of the returns is made on the upside on long trades rather than short trades, they tend to be more correlated on the downside, right?
Jagdeesh Prakasam:
And few of them master it absolutely but there are few or majority of them could not deliver on the downside in spite of being uncorrelated to the stock market. So in that sense, this is a case that is flipped over in that sense on the upside we really don’t care about it, but on the downside actually we wanted to be correlated. And you have a bigger suite of options on your hand than I do.
Jason Buck:
Yeah. We’re cheating, so it’s definitely. And then you also have that the interesting piece of that, like you kind of said before, is like on any sort of negative 10% move or less in the S&P, that’s a lot of noise and that’s not necessarily what any of us are hedging at. We were just sitting on that massive convexity that we can accelerate into those bigger down moves. And that’s what you can get out going along the VIX futures or buying put options. And that’s kind of the key to it is like you’re saying, if somebody long short, they don’t really have that convexity in a sell-off that’s why they tend not to do well in a sell-off, but we’re sitting on an inventory of very convex products that are going to dramatically outpace the sell-off in the S&P.
Jagdeesh Prakasam:
Absolutely.
Jason Buck:
And then so what I’ve got you here, so I’m going to cheat a little bit as well, is that we’re in the process of also building a basket of CTA trend following. And so you’re somebody who’s been in this space forever. So you’ve got to see how the space has changed and evolved. And as you said, you guys are working on random forest models for CTA trend, but it what’s even more interesting why I want to pick your brain about it is like, as you said, you guys were willing in 2008 even pivot away a little bit or reduce the exposure to manage futures. So you’re a bit agnostic about the space, but as you said, that’s coming back fully into your programs where you think it’s going to have a better future moving forward. Just in general, how have you seen the space evolve and how do you think about CTA trend or managed futures or trend following in general?
Jagdeesh Prakasam:
Yeah. So one of the classic… I mean, everybody, when we talk about trend following or managed futures, they think it’s a bunch of moving average crossover models that have been marketed really, really well. And that might have been the case in the past. As part of being in research at the time, I can attest that we did a lot of different things, which has nothing to do with moving average crossovers. But at the heart of it, fundamentally any model in the past, in the 2000s or in the ’90s was they used one data stream. So let’s say we were building a model for crude oil, we build the same model across all these markets. Okay. But the signal that the trade that we made on crude oil was always based on the data of crude oil alone, not on the data of any other markets.
Jagdeesh Prakasam:
So if there were influences of the S&P on crude oil or crude oil on S&P, or the dollar on S&P, that was never captured. It was all built off of one price series of the market itself. But then what we found is, especially after the financial crisis, there was a market structure change. There are multiple reasons for it. FED is definitely a big component of it, but also the rate of internet adoption and technology has been phenomenal in the past two decades. So you can only imagine that that has definitely caused a change in the market structure, right? And even now you see even with coming off of the cryptocurrency space taking off and actually all of these get reflected in the financial markets. Financial markets, I see it as the pulse of what the world is thinking right now. Anything happens, whether there is a terrorist attack or there is a pandemic, it gets reflected right there.
Jagdeesh Prakasam:
Or if there’s an election surprise, anything gets reflected in the financial markets, right? And what we found is these events are far more connected and far more broadcast and have an impact in the globalized world as we are. So there is actually a market structure change from where the decision tree models we’re doing well in the ’90s and 2000s, the world was not that connected. And today, guess what? Somebody has to eat a pangolin in another country and the rest of the world is shut down. It is one of those things. So we’re really connected from that standpoint. So there’s a huge market structure change here. So that’s part of that what we found is the trend itself, it’s gotten more complex. It is important to capture all the information that’s available to you.
Jagdeesh Prakasam:
So what we did is we came up with a framework that allows for that rather than a single decision tree just using the data from a given market, we said we’re going to take a portfolio of markets that’s there and get all the data information between these markets. So essentially we wanted to capture, is there a relationship between crude oil and S&P? Is there a relationship between the dollar and S&P? And if there is, we want to take that into our decision making process on putting on a trade on the S&P. So that framework, what we are using is random forests. We have created a framework in that sense and come up with an objective function. So when you build a random forest system, it’s important that you understand what is your objective.
Jagdeesh Prakasam:
The objective function is extremely important. So in our case, what we said is we have a long track record, whatever was our objective function throughout going back 30 years, we have kept that as constant. So when you see our 30 year track record, that’s a constant, but the methods used to arrive at that objective function could have evolved. And that’s exactly what we’re doing here. So in this particular place, our objective function is a signal to noise ratio. So what we’re saying is you can use a variety of ways of capturing signal to noise ratio. But essentially we are saying, if I wanted to go from point A to point B, what is the shortest possible line from point A to point B? How does it go? Is it really volatile? If it’s too volatile, the signal to noise ratio would be low for a market like that.
Jagdeesh Prakasam:
If it is smooth, the signal to noise ratio would be high. And what we’re saying is if we have all these different inputs coming in, influencers coming in, would we be able to achieve a smooth signal to noise ratio in the S&P in the next 20 days or 40 days? Whatever maybe the look back period in that metric. And so what we’ve done is… That’s a big part of it. And also this is a tad bit embarrassing going back 20 years when I used to build decision trees, there was a tendency to oh effect. And those days what we used to do is, oh, we got to do in sample and out of sample. That was sort of how we thought about the world. And what would happen is the funny thing is if your out of sample look bad, guess what? You’re going back to your model, tuning in back up until your out of sample look good.
Jagdeesh Prakasam:
So at that point, you would rather have the whole in and out of sample together because it probably makes your life easier if that’s what you’re trying to do, right? So we would always… It was a little bit subjective in terms of when we would stop doing that. So that was our way to kind of prevent overfitting. This is over 20 years ago, but we tried to do simulations where the alternate time series and things like that. We simulated different price paths so that the model would stay stable and that and this, but it was never satisfying because what would happen is some of these simulations would throw out price paths, which could not have happened or at least it didn’t look like it could ever happen.
Jagdeesh Prakasam:
And then the model if it falls apart there, we would say, well, that could never have happened. So I’m not really sure if I should take that into consideration. But one of the things with these frames… And also, I think the reason it worked, we did make good returns those years. The reason it worked was also one is of course, in trading, there’s always an element of luck. I can tell you that there’s a lot of elements of luck here, but the second part is also the market structure allowed for it. The market structure was fine, but in today’s market structure, it will not stand a chance. And that is what a lot of the CTA players are finding out. But what we’ve done differently is when you take a random forest and just to give you a feel for it’s very much related to decision trees.
Jagdeesh Prakasam:
So essentially let’s say we take 30 years of data, 200 trading days, 6,000 days. So you can think of 6,000 days of data and we can chop it up randomly to chunks of 200 or something. But we randomly pick it. So this is picked from the same distribution and we will fit one decision tree for each of these randomly picked samples. And ultimately what we are creating is weak learners. And what I mean by that is instead of taking one decision tree and doing it across 6,000 finds, we have taken that same decision tree and tried to optimize it only for those 200 fine pieces. They are weak learners. So we are distrustful of them. So we don’t actually trust any of them. And then we average it out. So it’s an ensembling technique that takes a bunch of weak learners and comes up with one decision.
Jagdeesh Prakasam:
And again, what we’re trying to do here is we’re looking for persistence and momentum. This is a momentum model, it’s a persistence and momentum. All we need is momentum to persist for another 20 days. We are not saying this is going to be the price or what have you. And the beauty of this framework is it’s very forgiving in the sense that let’s say there is a certain strong relationship between oil and the S&P and for a period of time that relationship flip, this will adapt to it. Or if there was another relationship that is picking up between the dollar and the S&P or the bonds and the S&P that will get… It allows for automatic pickup of those things while not putting too much weight on it, right?
Jagdeesh Prakasam:
So it gives it a vote, but it’s not completely tied to that. So you can’t go horribly wrong with it. So this ensembling technique kind of reduces the risk of overfitting. I like this way more than what I was doing 20 years ago. And admittedly, this is done by our very competent data science team and I’m fascinated with the ideas that they can come up with. And they’re far more intelligent than I am.
Jason Buck:
Well, basically it creates almost like a dimmer switch in that as each vote gets added to the system, it’s slowly changing the majority of those votes over time.
Jagdeesh Prakasam:
Correct.
Jason Buck:
And so it staggers into that position or that regime change or that market structure change, but you hinted at it earlier. What do you think about the market structure change of bonds moving forward? Let’s just say the classical trend followers have written this amazing bond bull market for the majority of their trading life. And if that market structure changed, as you hinted to, how do you think the ones that are just using classical decision trees are going to do?
Jagdeesh Prakasam:
They’re going to… I think it’s going to be a rough phase, okay? I think the jury is still out there in terms of what will happen to the bond market. I can tell you internally, we’ve been debating this saying, it feels like the FED comes in every time there is a little bit of a dip saying, buy the dip kind of a feeling. So we might still be in this sort of maybe a bond flat market or bond bull market for a few more quarters here. And there’s a strong opinion on the flip side of it. We’re saying, no, we are going to be in a better market. So it remains to be seen. But I think that is one of the risks. And that’s been seen even last year when if you saw the CTAs, many of the decision tree based CTAs hadn’t done well at all last year after Q1. And that kind of plays out. So it remains to be seen how they would do, or they may de-allocate to bonds. This might be a way for them to-
Jason Buck:
Like you said, in 2020 a lot of them would longer look back to a medium, they’ll longer look back Scott Whipsaw. They didn’t do well. They didn’t provide that crisis often in 2020, which is understandable. But what I’m more curious about too is what you hinted at earlier and just now, again, is like bonds may be in a certain proportion of their positions or maybe constrained bucket. But as you said, as the market shifted in 2008, a lot of those managed futures programs or those CTA programs to stay relevant and to raise AUM, not only one, did they reduce volatility targeting because that’s what people wanted, but the other thing is they transitioned more to the financial side of the equation and more trend following those markets, and a lot of them kind of reduced, or it got rid of those commodity exposure positions.
Jason Buck:
So how do you guys think about internally? Do you have a band of framework or a band of percentages that you still hold in commodities knowing sure we may not have seen a lot of commodities in the last decade, but that could turn around, that market structure could change relatively quickly into the future?
Jagdeesh Prakasam:
Right. So what we’ve done is that is… I had multiple opinions. I mean, I have a different opinion on this because, it’s highly risky in my opinion to add an additional layer of parameters. So when you add this dynamic algorithm to weight the sectors differently, there’s an additional layer of parameters that you’re tuning to. If you get it right, good for you, if not, you’re in trouble. So a lot of the CTAs did that even with equities. After 2011 and 2012, when they had two down years, they decided that they were going to turn up the waiting a little bit more to the equity sector. And some of them picked up on that alpha. On that, I didn’t say alpha, but would have picked up on the returns there, right? But when during crashes they were not able to provide the same level of diversification that you would have expected from them. So that’s the challenge.
Jagdeesh Prakasam:
So what we have done is we keep a 25% equal risk budgeting across all the four sectors. So if there is a movement, if there’s a new market regime, there is enough of a risk budget available to go after it. And we also have a risk budget on a market by market level. So we don’t … It’s a margin to equity risk budget, but we kind of keep that so that we don’t get highly concentrated in few markets even though we are trading 80 plus markets. So that’s something… That’s how we view it. If you’re trying take out, what we try to do is we really cared about overfitting, we’re really scared. We lose sleep over overfitting, right? So we always want to make sure that we are trying to not overfit in any way. So what we’ve done is we’ve tried to eliminate as many unwanted parameters as possible. And as part of that, we’ve kept this as an equal risk budget rather than tuning that.
Jason Buck:
Okay. So I’ve monopolized more of your time than I said I would, but before I ask you where people can find you some people may have heard of Rotella or maybe not, but then they’ve heard of Qdeck or vice versa. So what’s Qdeck in the relationship with Rotella?
Jagdeesh Prakasam:
So Qdeck is our FinTech product. So what we are trying to do with Qdeck is what I would say Starbucks did with the coffee industry, with mass customization. So that is our goal with Qdeck. It’s a hedge fund. It’s a service model. What we believe is ultimately investors want solutions to fit their exposures. And this is a way for them to come in and build portfolios to come up with custom solutions to their various needs. So on that front, we do have a couple of clients using it right now. We have actually opened it up to the RIA world as well. So for the RIAs actually, we are building robo-advisors for them so that they can efficiently use some of our machine learning knowledge and build ideas that could potentially compete very well with the existing robo-advisor offerings that are there out in the market. So that’s something… That’s what’s Qdeck. So Qdeck is very much part of Rotella and it’s a FinTech product that is managed by our data science team.
Jason Buck:
And where should people go if they want to learn more about Qdeck or Rotella?
Jagdeesh Prakasam:
Qdeck.com. And it has information on both Rotella as well as Qdeck. Yeah.
Jason Buck:
Perfect. Thank you so much for your time, Jag. I really appreciate you coming on the podcast.
Jagdeesh Prakasam:
Yeah. Thanks a lot, Jason. Thanks for your invitation.
Taylor Pearson:
Thanks for listening. If you enjoyed today’s show, we’d appreciate if you would share this show with friends and leave a short review on iTunes as it helps more listeners find the show and join our amazing community. To those of you who’ve already shared or left a review, thank you very sincerely. It does mean a lot to us. If you’d like more information about Mutiny Fund, you can go to mutinyfund.com. For any thoughts on how we can improve the show or questions about anything we’ve talked about here on the podcast today, drop us a message via email, I’m Taylor@mutinyfund.com and Jason is Jason@mutinyfund.com. Or you can reach us on Twitter, I’m @TaylorPearsonMe and Jason is @JasonMutiny. To hear about new episodes or get our monthly newsletter with reading recommendations, sign up at mutinyfund.com/newsletter.